What is predictive maintenance of clinical analysis machines?
Today’s diagnostic machines are riddled with sensors. These sensors provide a multitude of prescriptive information to know: the number of times you have used it per day to make diagnoses, the amount of reactive fluid you have to make the tests and also how much reactive fluid is left (knowing how many tests you can make before the fluid runs out), the state of the machinery, parts with a higher rate of wear…
In short, nowadays you can find out what state a clinical analysis machine is in remotely as long as it is connected.
How is this monitoring of diagnostic machines carried out? The reality is that thanks to the 3G and 4G, we can now have almost real time information about any diagnostic device that is connected. When we talk about the data generated by the clinical analysis machines, we are not talking about large volumes of data, but instead we are talking about prescriptive information for decision-making (being able to anticipate the purchase of reactive liquid before it runs out, being able to estimate the volume of patients etc).
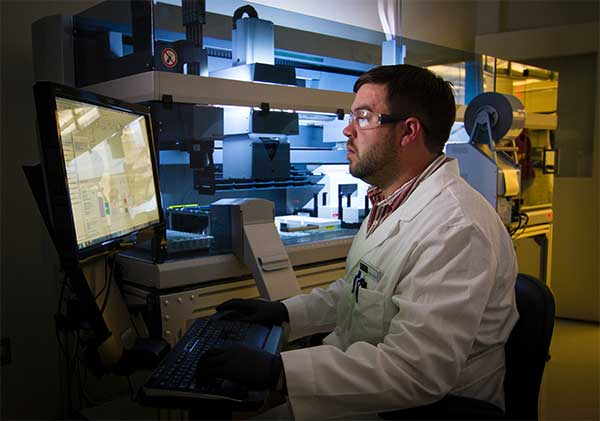
If we look exclusively at the theoretical part, predictive maintenance is going to evaluate the state of the machinery making recommendations to intervene or not depending on its condition. The truth is that predictive maintenance means great savings for companies.
Advantages of predictive maintenance
When we compare predictive maintenance with corrective maintenance, the advantages of predictive maintenance become more than evident.
This type of maintenance allows us to get a better performance from the clinical analysis machine we are monitoring, avoiding also having to make major repairs, dismantling and adjustments. As a consequence, all this will extend the life of the machine thanks to making small adjustments (lubrication, re-tightening, alignment, replacement of reactive liquid, etc).
Another thing to bear in mind is that predictive maintenance is carried out “on-condition”, i.e. with the machine running. In this way, patient care does not have to be stopped and waiting time does not have to be extended.
Something to bear in mind is that when predictive maintenance is applied we will be able to detect breakdowns in their most initial stage. In this way, there will be room to carry out maintenance and correction actions, etc., in a programmed way, outside the diagnostic peaks. This will further optimize the cost of repair.
When the failure of a part is anticipated, we will be having the opportunity to buy spare parts avoiding the storage stock (or what means immobilized capital for a company). This will ensure that we have the part we need at just the right time.
You could say that predictive maintenance is, in a way, proactive maintenance, since it allows us to carry out repairs before critical machine failures occur (as opposed to reactive maintenance).
Predictive maintenance to improve the efficiency of diagnostic machines
The truth is that predictive maintenance allows us to take operational efficiency further. Based on the accumulated telemetry history we can look for condition-based maintenance strategies. It is about predicting failures of critical elements of the diagnostic machine before they occur and planning their replacement in advance, while extending the life of the parts. For predictive maintenance we need to use machine learning techniques to obtain failure prediction models. These predictive models will be fed by real-time telemetry to identify deterioration and faults in advance. The models operate on time series of a wide variety of physical magnitudes and other signals.
The challenge of predictive models in diagnostic machines
Getting a predictive model of the condition of an element of a diagnostic machine is often a challenge no less. It is easy to train a supervised learning algorithm when you have a large fault history, but that is not realistic.
The reliability of diagnostic machines means that fault monitoring occurs very infrequently, so that the data set is not viable. Particularly important in this case are unsupervised approaches and algorithms that learn from normality to infer when a relevant deviation begins to occur that can end in a serious fault.