Technology using artificial intelligence (AI) continues to advance by leaps and bounds and is rapidly becoming a potential disruptor and essential enabler for almost all businesses in all sectors. Today, one of the barriers to the widespread deployment of AI framework is no longer the technology itself, but a set of challenges that are ironically much more humane: ethics and human values.
AI that discriminate on the basis of race, age or gender
As AI expands in almost every aspect of modern life, the risks of misusing AI increase exponentially to a point where those risks can literally become a matter of life and death. Some of the great examples of AI that have “broken down” include systems that discriminate against people because of their race, age or gender and social media systems that inadvertently spread rumors and misinformation and more.
But know that these examples are only the tip of the iceberg. As AI is deployed on a larger scale, the associated risks are likely to only increase, which could have serious consequences for society at large, and even greater consequences for responsible businesses. From a business perspective, these potential consequences range from lawsuits, regulatory fines and angry customers to embarrassment, reputation damage and destruction of shareholder value.
Having artificial intelligence, which is essential for companies
However, with AI now becoming a core business differential, not just something desirable for companies.
Companies must learn to identify and manage AI risks effectively. To achieve the potential for collaboration between people and machines, organisations must communicate a plan for AI that is adopted and discussed by the company’s own board of directors.
AI Trust Framework
Deloitte’s trusted AI framework introduces six key dimensions that, considered collectively in the design, development, deployment and operational phases of implementing the AI system, can help safeguard ethics and build a trusted AI strategy.
The trusted AI framework is designed to help companies identify and mitigate potential risks related to the ethics of AI at each stage of the AI life cycle. Each of the six dimensions of the framework is examined more closely below.
Fair, Unbiased
A trustworthy AI must be designed and trained to follow a fair process, consistent with making fair decisions. It should also include internal and external controls to reduce discriminatory bias.
Bias is an ongoing challenge for humans and also for society, not only for AI. However, the challenge is even greater for AI because it lacks a nuanced understanding of social norms – not to mention the extraordinary general intelligence needed to achieve “common sense” – which can lead to decisions that are technically correct but socially unacceptable. An AI learns from the datasets used to train it, and if those datasets contain real-world biases, AI systems can learn, amplify and propagate that bias at digital speed and scale.
For example, an AI system that decides on the fly where to place job advertisements online might unfairly direct advertisements for higher-paying jobs to male visitors to a website because real-world data show that men tend to earn more than women. Similarly, a financial services company that uses artificial intelligence to screen mortgage applications might find that its algorithm unfairly discriminates against people based on factors that are not socially acceptable, such as race, gender or age. In both cases, the company responsible for the AI could face significant consequences, such as regulatory fines and reputational damage.
To avoid issues of fairness and bias, companies should first determine what constitutes “fair”. This may be much more difficult than it appears, as for any given issue there is generally no single definition of “fair” on which everyone agrees. Companies also need to actively search for bias within their algorithms and data, making necessary adjustments and implementing controls to help ensure that additional bias does not arise unexpectedly. When a bias is detected, it needs to be understood and then mitigated through established processes to resolve the problem and restore customer confidence.
An AI can no longer be treated as a “black box” that receives inputs and generates outputs without a clear understanding of what is going on inside.
Transparent and easy to explain
For AI to be reliable, all participants have the right to understand how their data are being used and how the AI is making decisions. The algorithms, attributes and correlations of AI must be open to inspection, and their decisions must be fully explainable.
For example, online retailers that use AI to make product recommendations to customers are under pressure to explain their algorithms and how recommendation decisions are made. Similarly, the United States judicial system is faced with ongoing controversy over the use of opaque AI systems to inform decisions about criminal judgments.
Important issues to consider in this area include identifying cases of AI use for which transparency and explainability are particularly important, and then understanding the data that are used and how decisions are made for those use cases. In addition, with regard to transparency, there is increasing pressure for people to be explicitly informed when they interact with AI, rather than for AI to be disguised as a real person.
Accountability
Reliable AI systems should include policies that clearly establish who is responsible and accountable for their results. Blaming technology itself for bad decisions and miscalculations is not enough, either for the people who are harmed or, of course, for government regulators. This is a key issue that is only likely to become more important as AI is used for an increasingly wide range of increasingly critical applications, such as disease diagnosis, wealth management and autonomous driving.
For example, if a vehicle without a driver causes a collision, who is responsible and accountable for the damage? The driver? The vehicle owner? The manufacturer? The AI programmers? The CEO?
Similarly, consider the example of an investment firm using an automated AI-driven platform to trade on behalf of its clients. If a customer invests his life savings through the firm and then loses everything because of poor algorithms, there should be a mechanism to identify who is responsible for the problem, and who is responsible for doing things right.
Key factors to consider include laws and regulations that could determine legal liability and whether artificial intelligence systems are auditable and covered by existing whistleblower laws. In addition, how will problems be communicated to the public and regulators, and what are the consequences for responsible parties?
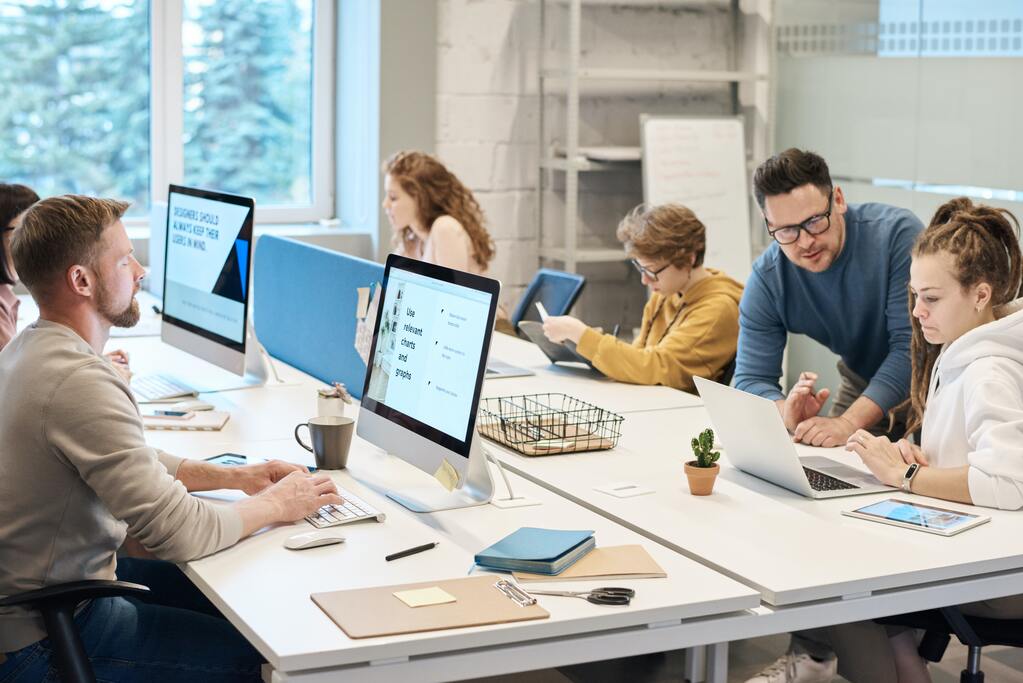
Robust and reliable
For AI to achieve widespread adoption, it must be at least as robust and reliable as the traditional systems, processes and people it is augmenting or replacing.
For AI to be considered trustworthy, it must be available when it is supposed to be and must generate consistent and reliable results that adequately perform tasks under less than ideal conditions and when unexpected situations and data are encountered. Reliable AI must scale well, remaining robust and reliable as its impact expands and grows. And if it fails, it must fail in a predictable and expected manner.
Consider the example of a healthcare company using AI to identify abnormal brain scans and prescribe appropriate treatment. To be reliable, it is absolutely essential that AI algorithms produce consistent and reliable results because lives could be at stake.
To achieve robust and reliable AI, companies need to ensure that their AI algorithms produce the right results for each new data set. They also need established processes to handle problems and inconsistencies that may arise. The human factor is a critical element in this regard: understanding how human input affects reliability; determining who the right people are to provide the input; and ensuring that those people are properly equipped and trained, particularly with respect to bias and ethics.
Privacy
Privacy is a critical issue for all types of data systems, but it is especially critical for AI, as the sophisticated knowledge generated by AI systems often comes from more detailed and personal data. Trustworthy AI must comply with data regulations and only use the data for the stated and agreed upon purposes.
The issue of AI privacy often extends beyond a company’s own walls. For example, the privacy of audio data captured by AI assistants has made headlines in recent times, and controversies have arisen over the extent to which data is accessible to a company’s suppliers and partners, and whether it should be shared with law enforcement agencies.
Companies need to know what customer data is being collected and why, and whether the data is being used in the way that customers understood and agreed to. In addition, customers should be given the required level of control over their data, including the ability to opt in or out of having their data shared. And if clients have concerns about data privacy, they need an avenue to express those concerns.
Security and protection
To be trustworthy, AI must be protected from cyber-security risks that can lead to physical and/or digital damage. While security and protection are clearly important for all IT systems, they are especially crucial for AI due to the increasing role and impact of AI on real-world activities.
For example, if an AI-based financial system is hacked, the result can be reputational damage and loss of money or data. These are serious consequences, of course. However, they are nowhere near as serious as the possible consequences of an AI-driven vehicle being hacked, which could endanger people’s lives.
Another example of AI cyber security risk is a recent data breach involving millions of fingerprint and facial recognition records. This breach was particularly serious because it involved biometric data of individuals, which are permanent and cannot be altered (unlike a stolen password or other standard data that can be quickly and easily changed to limit damage).
Can an AI be trusted?
The ethics of AI are emerging as the single greatest challenge to the continued progress of AI and its widespread deployment, and it is a challenge that companies can no longer ignore now that AI is becoming a core business capability. The trusted AI framework provides a structured and comprehensive way of thinking about the ethics of AI, helping companies to design, develop, deploy and operate AI systems they can trust.