Success in the business world lies in the ability to extract valuable insights from the data around us. Computer vision applications is leading this transformation, enabling companies not only to collect visual data but also to use it for smarter and more efficient decision-making. From pattern detection to process automation, computer vision is redefining how businesses operate.
Its applications are making a significant impact across various industries. Smart cameras, powered by advanced algorithms, can detect defects with greater precision than the human eye. These innovations are opening new opportunities for growth and transformation in multiple sectors.
In this article, I’ll explore how these applications alongside AI and IoT integration are paving the way for a future where computer vision becomes an indispensable tool for businesses across all industries.
Generative AI: A boost for computer vision
Generative Artificial Intelligence (Gen AI) is an exciting branch of AI focused on creating entirely new data, such as images, videos, or even text, based on existing information. By leveraging machine learning algorithms and neural networks, Gen AI learns patterns and structures within data, becoming a creative force in computer vision. This powerful combination is revolutionising both technology and creativity, reshaping how we perceive and interact with data. How does it achieve this? Let me explain:
Creation of innovative visual content
The creation of innovative visual content has become a crucial area for businesses looking to stand out in the market. Generative Adversarial Networks (GANs) have emerged as a key tool in this field, enabling the generation of highly realistic images from existing data. These models pit two neural networks against each other one generating images and the other evaluating them fostering competition that results in increasingly convincing visuals.
Meanwhile, autoencoders offer the ability to generate image variations or even create content from textual descriptions, providing businesses with greater creative flexibility. Additionally, diffusion models allow for fine control over the generation process, transforming random noise into realistic images step by step.
Understanding and analysing images
Understanding and analysing images is essential across various business sectors, from product recognition to interpreting complex visual data. Convolutional Neural Networks (CNNs) have become a cornerstone in this field, enabling the extraction of features and patterns from images for a deeper understanding of visual content.
Attention mechanisms are another key tool, allowing neural networks to focus on specific areas of an image, thereby improving the accuracy and relevance of analysis. Lastly, transfer learning leverages pre-trained models to accelerate the learning process and enhance AI performance in computer vision tasks.
Multimodal AI: The future of computer vision?
Multimodal AI has introduced a new paradigm for understanding and analysing the visual world. Unlike traditional approaches that focus on a single type of sensory data, such as images or videos, multimodal AI integrates information from various sources images, videos, audio, text, and sensor data to achieve a more comprehensive understanding.
This robust approach has significant implications for business decision-making, product and service enhancement, and process optimisation across various industries, from healthcare to security and entertainment.
By fully leveraging machine learning algorithms, multimodal AI can support business planning by recognising diverse types of information and providing more precise and detailed insights. By combining data from multiple streams, it can predict financial outcomes and even anticipate maintenance needs. For instance, if ageing equipment is not receiving the necessary attention, a multimodal AI-powered computer vision system may infer that it requires less frequent maintenance, helping to reduce unnecessary costs and downtime.
Computer vision with edge and lightweight architectures
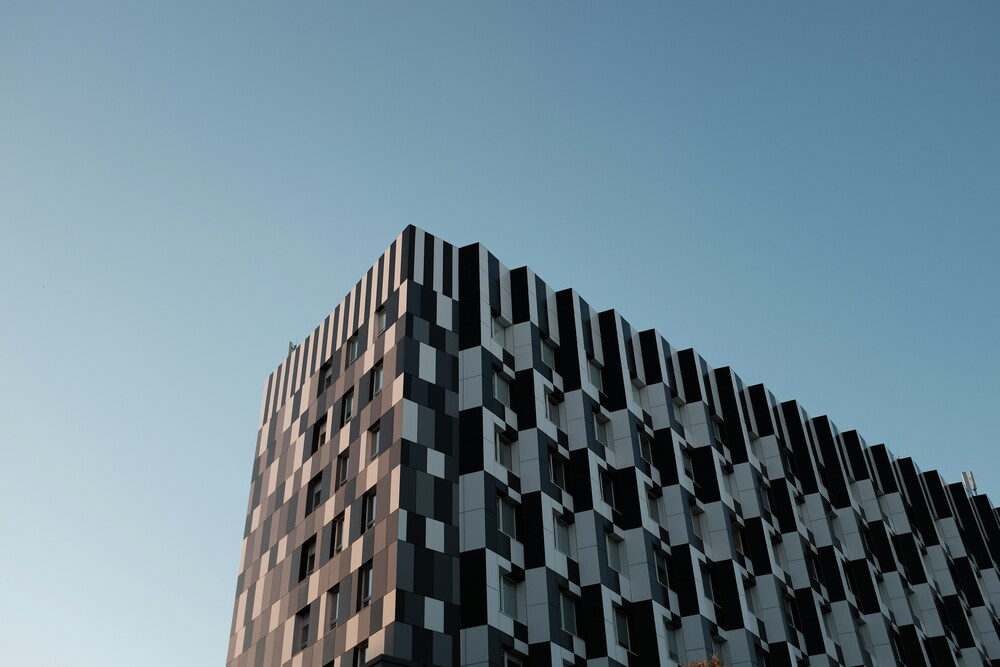
Computer vision with Edge and lightweight architectures is transforming the field of real-time computer vision, ushering in a new paradigm for processing and analysing visual data.
Unlike traditional approaches that centralise data processing on remote servers, Edge computing enables data to be processed directly on the device or sensor collecting it, reducing latency and enhancing privacy.
Impacts of computer vision with edge
For industries with needs for process automation and improvements in operational efficiency, integrating computer vision with Edge enhances the interaction between humans and computers, generative AI, and traditional machine learning solutions for informed decision-making.
In this business environment, the implementation of technologies such as computer vision with Edge provides a range of key benefits.
Agility is one of them: by processing data directly on the device, errors are reduced, and response times are shortened, expanding production capacity and enabling more business to be managed with the same available resources.
Furthermore, in terms of the shopping experience, the use of data enhances customer interactions, driving retention and increasing the lifetime value (LTV) of each customer.
Finally, regarding security, Edge computing allows for the identification of risk situations, such as detecting intruders in restricted environments, anticipating potential problems, and enabling an immediate response, thus ensuring a safer environment for business operations.
Impacts of lightweight architectures
Lightweight architectures are essential for Edge computing, as they enable the execution of complex computer vision models on devices with limited resources. These architectures are based on techniques such as convolutional neural networks (CNNs), which are highly efficient for processing images and videos, and can be implemented on devices with low processing power.
Moreover, on-device machine learning allows for training and executing models directly on the device, without the need to send data to a central server.
Distributed computing also plays a crucial role by enabling the distribution of data processing across multiple devices, thus improving the performance and scalability of the system.
Satellite computer vision
Satellite computer vision is a key technology for businesses that need to monitor and analyse the environment from a global perspective. With this tool, it is possible to track land use, detect climate changes, and gather detailed information about natural resources. This type of analysis is essential for resource management, agriculture, urban planning, and environmental research.
In terms of resource management, satellite computer vision provides precise data on land distribution and usage, enabling strategic decisions regarding its conservation and sustainable use.
In the agricultural sector, these images help monitor crops, identify plant health issues, and predict yields, optimising agricultural processes and increasing productivity.
In the urban realm, this technology is crucial for planning city growth, identifying areas vulnerable to natural disasters, and evaluating urban policies.
Finally, in environmental research, satellite images allow the study of phenomena such as deforestation, climate change, or air and water quality, providing valuable information for business decision-making focused on sustainability and environmental responsibility.
Application of AI technologies in satellite images
Some common examples of computer vision techniques that can be applied to aerial images include object detection in images using techniques such as YOLO, image segmentation into its key components with instance segmentation, image classification into different categories, and feature matching to detect if two different images have captured the same object.
These tools provide businesses with a wide range of capabilities to analyse and leverage data collected by satellites, which can lead to more informed and strategic decision-making.
Use case: Computer vision + environmental efficiency
Shell, in its pursuit of innovative solutions for environmental conservation, has employed computer vision techniques, demonstrating it to be an invaluable tool. Through the Shell Gamechanger project in Brazil, the company is using geo-tagged social media images to enhance the interpretation of satellite imagery and ground sensor data.
By leveraging images voluntarily shared by social media users, the health of ecosystems is assessed. With the help of AI, the visual world is interpreted and understood through untapped data, such as images on social networks.
AI can provide crucial insights into how ecosystems function as carbon sinks and how they may change over time.
This initiative demonstrates that computer vision not only complements on-site inspections but also significantly boosts confidence in the effectiveness of nature-based solutions (NbS) projects.
By employing these advanced technologies, companies can improve their conservation strategies and contribute more effectively to environmental preservation.
Computer vision in the fight against digital misinformation and cybersecurity
Deepfake detection is a crucial technology for protecting the integrity of online information and ensuring cybersecurity. It involves identifying and removing digitally manipulated content, such as fake images or videos, which can damage the reputation of individuals and businesses, as well as spread misinformation.
By using advanced computer vision algorithms and machine learning, deepfake detection analyses multimedia content to identify any signs of manipulation. This is essential for safeguarding a company’s reputation, especially in a digital environment where misinformation can harm customer trust and brand credibility, and where cybersecurity is increasingly relevant in the protection of data and digital assets.
To address these threats, deepfake detection has become a rapidly growing field:
Detection techniques
- Facial analysis: These techniques can identify inconsistencies in facial expressions, head movements, or blinking, which could indicate manipulation in multimedia content.
- Audio analysis: By using audio analysis techniques, irregularities in the voice can be detected, such as changes in tone, speed, or pronunciation, which could suggest manipulation in audio recordings.
- Motion analysis: These techniques allow the detection of discrepancies in body movement, such as synchronisation with audio or realistic physics, signs that may indicate manipulation in videos.
- Pattern analysis: This technique focuses on identifying unusual patterns in images or videos, such as discrepancies in lighting, textures, or reflections, which could suggest that the content has been altered.
Applications of deepfake detection
There are several sectors where deepfake detection is particularly important:
Media: The media heavily relies on the credibility of its content. Deepfake detection in images and videos helps the media verify the authenticity of news and prevent the spread of misinformation. This is crucial for maintaining public trust and preserving the integrity of journalism.
Government agencies: Governments use computer vision to detect deepfakes and combat disinformation, especially in the context of national security. The ability to identify manipulated content in real-time is essential for protecting the public from potential threats and preserving political stability.
Final Reflection
Computer vision will play a pivotal role in the future of businesses and society as a whole, thanks to the continuous advancement of machine learning algorithms, particularly in the field of deep learning. With this ever-evolving technology and the increasing availability of visual data, it will become an indispensable tool for a wide range of business applications.
From fraud detection to optimising manufacturing processes, the ability to analyse vast amounts of visual data quickly and accurately will enable more informed and efficient decision-making, resulting in increased productivity and profitability. In the social sphere, deep learning will enable significant improvements in key areas such as healthcare, security, and transport.
If you’re still not convinced of this reality, we invite you to discover how Opensistemas is implementing it or how other companies in Spain are applying it. After this, if you still have doubts or already see the significant impact it could have on your processes and are certain it’s what you need, get in touch with our team of experts. Remember, if you’re a company looking for a complete change, not just in your processes but also to improve your market position, this technology can change, innovate, transform, and reduce costs; it’s exactly what any business needs.