Behavioural analysis and prediction of customer churn
Would it be possible to predict when our customers will leave us? Predicting the behaviour of our clients by studying customer churn is one of the areas to which companies devote most attention and resources. If we know how a client is going to act and, more importantly, at what stages of the process – from their interest to the purchase or acquisition of our service – they may lose interest in, we can act to cover those shortfalls and improve as a company.
Management of customer turnover
All companies in the consumer market and business sectors have to deal with customer turnover, as it is a key factor in estimating the company’s revenue and therefore influencing sales. Furthermore, an increase in customer retention is equivalent to a more than proportional reduction in costs, as acquiring new customers is much more costly than retaining existing ones.
The objective of studying customer rotation is not based on looking for faults in our product or our team, but on creating a strategy to improve customer retention. To do this, we will study customer behaviour, transactions, demographics and usage patterns.
For this purpose, we can use churn models, i.e. predictive models that calculate the customer churn rate. The effective implementation of these models in several customer segments will help us reduce churn and promote retention.
The solution: Knowledge Graphs and xAI, explainable artificial intelligence
Currently, thanks to graph technology, better known as Knowledge Graphs, and to xAI, explainable artificial intelligence, we can improve the predictive capacity of the existing churn models incorporating social dynamics such as relationships or customer communication flows.
This solution captures social relation dynamics in knowledge graphs in the residential market. This helps to improve the churn model with new features using relationship and motif graph embeddings.
Benefits of using a customer churn model
The use of these models will increase the predictive potential using graphs that represent social relationships. Social graphs are very useful, since we can monitor if there are variables that cause a client to end his relationship with the company. If his friends or family leave our organization it can also increase the probability of our client leaving.
Thanks to this, we have a long prediction horizon to prevent turnover, as well as boosting customer acquisition from existing customer relationships.
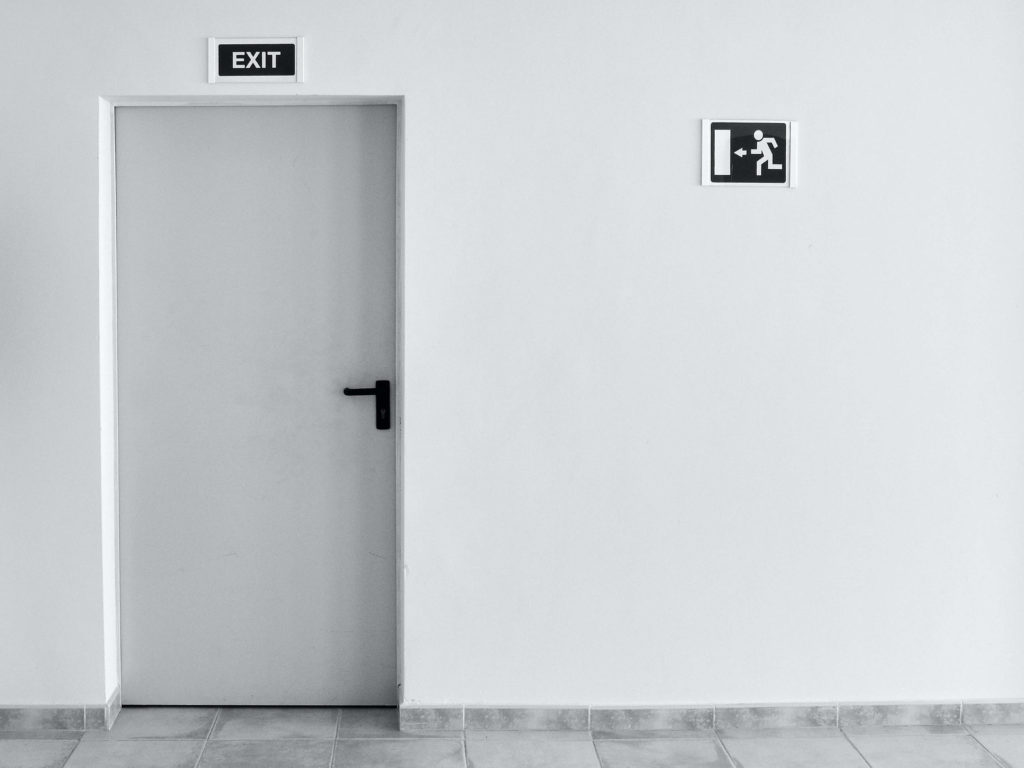