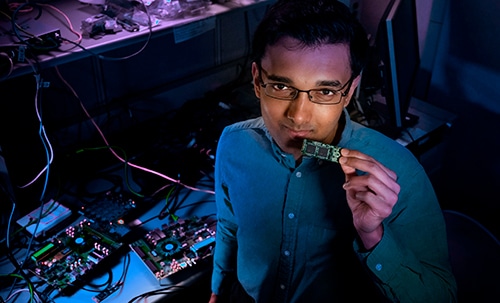
Our brain can do lots of things, but the way it helps us smell is one of the best understood. When a smell reaches the olfactory cells in our nose, they send a signal to the corresponding group of brain neurons known as the olfactory bulb. The bulb then transports the signal to other parts of the brain, allowing us to appreciate the perfume of a grapefruit or avoid the stench of garbage.
Olfactory bulbs are specific to mammals, but other animals, such as insects, also exhibit similar neural structures. This means that “there is probably something quite fundamental and efficient about these implementations if evolution has reached them in different cases,” says Mike Davies, the director of Intel’s Neuromorphic Computing Laboratory.
Both because they’re so efficient and because we understand them so well, olfactory systems are a great starting point for neuromorphic chips, a new type of computer hardware that is inspired directly by the structure of the brain.
Neuromorphic chips that allow computers to be smelled
On Monday, Intel scientists published a paper in Nature that proposes a new neuromorphic chip design that mimics the structure and capabilities of the olfactory bulb. The researchers worked with olfactory neurophysiologists who study the brains of animals as they smell. They designed an electrical circuit, based on the neural circuits that are activated when their brains process an odor, that could be carved into a silicon chip. They also designed an algorithm that reflects the behavior of electrical signals pulsing through the circuit. When they trained the algorithm on the chip using an existing data set of 10 “odors” – characterized by their measurements from 72 different chemical sensors – it was able to accurately distinguish them with far fewer training samples than a conventional chip.
This chip is still a prototype at a relatively early stage, but once mature it could be used for various applications, such as bomb detection or detection of noxious fumes in chemical plants. It also demonstrates the potential of neuromorphic computing for more data-efficient AI.
Deep learning algorithms
Today, the most popular chips for running state-of-the-art deep learning algorithms follow a von Neumman architecture, a design convention that has driven the computing revolution for decades. But these architectures are inefficient for learning: the algorithms running on them require massive amounts of training data, in contrast to our much more efficient brains. Neuromorphic chips, therefore, try to preserve the structure of the brain as much as possible. The idea is that such close imitation will increase the learning efficiency of the chip. In fact, Intel got the chip to learn from very few samples.
In the future, the research team plans to improve the design of its neuromorphic chip and apply it to other brain functions beyond smell. Davies says the team will likely focus on vision or touch next, but has long-term ambitions to address more complex processes. “Our sensory mechanisms are the natural place to start because they are well understood,” he says. “But in a sense we’re working on the brain, even the higher order thought processes that happen.”